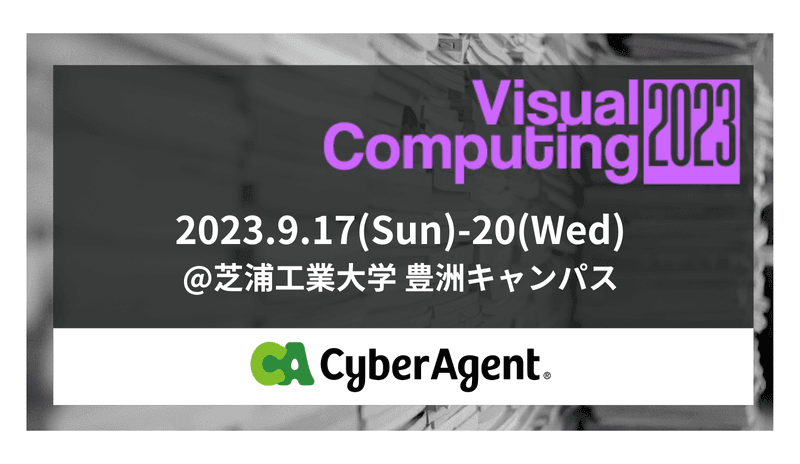
Visual Computing 2023について
今年で開催31回目を迎える、産学の垣根を超えて技術者・研究者・クリエイターが交流する、コンピュータ・グラフィックスおよび周辺分野における国内最大規模のシンポジウムです。
Visual Computing 2023において株式会社サイバーエージェントは、スポンサー講演・論文セッション・ポスター発表・企業展示など発表を行うほか、新設された「極(きわみ)AIお台場スタジオ」での全体懇親会も開催いたします。
サイバーエージェントAI Labのメンバーだけで取り組んでいるものだけでなく、産学連携先と進めている研究内容となっています。
民間企業の研究組織がどのようなデータで、どのような研究を行っているのか、興味をお持ちの方はぜひ発表にお越しください。
参加希望の方はこちらからご登録下さい。
【スポンサー講演】
日時 | 9月18(月) 10:00 - 10:20 スポンサーセッション |
タイトル | サイバーエージェントにおけるCG技術への取り組み |
講演者 | 櫻井 快勢 (AI Lab リサーチサイエンティスト) |
概要 | 事業例や研究例を中心に、インターネット広告事業を軸としたCGに関する取り組みをご紹介します。 |
【口頭発表・招待講演プログラム】
日時 | 9月18日(月) 14:10-15:00 国際招待セッション1: フェイスとエディティング |
タイトル | [13] BlendFace: Re-designing Identity Encoders for Face-Swapping |
著者 | (東京大学) 塩原 楓 氏 (AI Lab リサーチサイエンティスト) 楊 興超 武富 貴史 |
概要 | The great advancements of generative adversarial networks and face recognition models in computer vision have made it possible to swap identities on images from single sources. Although a lot of studies seems to have proposed almost satisfactory solutions, we notice previous methods still suffer from an identity-attribute entanglement that causes undesired attributes swapping because widely used identity encoders, eg, ArcFace, have some crucial attribute biases owing to their pretraining on face recognition tasks. To address this issue, we design BlendFace, a novel identity encoder for face-swapping. The key idea behind BlendFace is training face recognition models on blended images whose attributes are replaced with those of another mitigates inter-personal biases such as hairsyles. BlendFace feeds disentangled identity features into generators and guides generators properly as an identity loss function. Extensive experiments demonstrate that BlendFace improves the identity-attribute disentanglement in face-swapping models, maintaining a comparable quantitative performance to previous methods. |
日時 | 9月18日(月) 16:20-17:50 ポスターセッション1 |
タイトル | [P28] 顔パーツの指定によるアニメ調イラストからの3D顔モデルの半自動生成 |
著者 | (法政大学) 秋元 優希 氏 (法政大学/プロメテックCGリサーチ) 佐藤 周平 氏 (AI Lab リサーチサイエンティスト) 櫻井 快勢 |
概要 | 本研究では,アニメ調の顔のイラストから半自動で顔の3Dモデルを生成する手法を提案する。顔の正面と側面の2枚のイラストを用意し、それぞれにおいて顔パーツが存在する矩形範囲と目の領域をユーザは指定する。それを基にして得られたパーツの領域の正面と側面の対応から3次元空間上に点を生成し、できた点群に対してボールピボットアルゴリズムを用いて面を生成する。 |
日時 | 9月19日(火) 10:00-10:50 セッション6: 変形 |
タイトル | [27] Mode-Decomposed Neural Radiance Field for Motion Synthesis (long) |
著者 | (大阪大学) Shengze Zhong 氏 (AI Lab リサーチサイエンティスト) 武富 貴史 |
概要 | We model the motion of the dynamic neural radiance field in the frequency domain for motion synthesis. |
日時 | 9月20日(水) 10:50-11:35 セッション9: デジタルヒューマン |
タイトル | [43] 連続的パラメータによる顔画像の化粧制御 (long) |
著者 | (AI Lab リサーチサイエンティスト) 楊 興超 武富 貴史 (筑波大学) 遠藤 結城 氏 金森 由博 氏 |
概要 | 本研究は、顔写真の化粧の濃淡という直感的な属性を連続的パラメータにより調整する技術を提案する。提案手法では、化粧の注目度が高い領域であるアイシャドウと口紅の編集に着目する。主な貢献は次の4点である。(1) 編集対象領域の手動での選択が不要、(2) 入力画像の化粧に依存しない幅広い化粧の濃淡調整、(3) 低い計算コスト、 (4) 化粧の濃淡の手動アノテーションが不要なデータセットの作成。 |
<関連リンク>
VC2023公式サイト:https://visualcomputing.jp/vc2023/
AI Lab 公式サイト:https://cyberagent.ai/ailab/
株式会社Cyber AI Productions:https://www.cyber-ai-productions.co.jp/